Federated Cybersecurity: Collaborating Without Sharing Data
- Swarnali Ghosh
- 21 hours ago
- 6 min read
SWARNALI GHOSH | DATE: MAY 09, 2025
Introduction
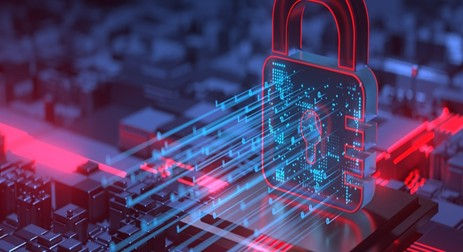
In an era where data privacy is paramount and cyber threats are increasingly sophisticated, organizations face the challenge of enhancing their cybersecurity measures without compromising sensitive information. Federated learning (FL) emerges as a revolutionary approach, enabling collaborative cybersecurity efforts without the need to share raw data. In an era where cyber threats are growing in sophistication and scale, organizations face a critical dilemma: how to collaborate on cybersecurity without compromising sensitive data. Traditional methods of sharing threat intelligence often require exchanging datasets, which can expose proprietary information or violate privacy regulations. Enter federated cybersecurity—a revolutionary approach that enables organisations to strengthen their defences without directly sharing data.
Understanding Federated Learning in Cybersecurity
Federated learning is a decentralized machine learning technique where multiple entities (clients) collaboratively train a shared model while keeping their data localised. Instead of transferring raw data to a central server, each client processes data locally and shares only model updates, such as gradients or parameters. This approach ensures data privacy and security, making it particularly suitable for cybersecurity applications where sensitive information is involved. In the context of cybersecurity, FL allows organisations to collectively improve threat detection models by learning from diverse datasets without exposing proprietary or confidential information. This collaborative intelligence enhances our ability to detect and respond to cyber threats more effectively. Federated cybersecurity is a collaborative security framework where multiple organisations collectively analyse threats and improve defences without exchanging raw data. Instead of pooling datasets into a central repository, this model relies on decentralized computation, allowing participants to derive insights while keeping their data private.
Key Principles of Federated Cybersecurity
Data Privacy Preservation: Organisations retain control over their data, sharing only aggregated insights or anonymised metadata.
Decentralised Analysis: Threat intelligence is processed locally, with only results (not raw data) shared across the network.
Machine Learning & AI Integration: Federated learning allows AI models to be trained across multiple sources without direct data exposure.
Regulatory Compliance: Helps organisations adhere to GDPR, HIPAA, and other data protection laws by minimising data transfers.

This approach is particularly valuable in industries like finance, healthcare, and critical infrastructure, where data sensitivity is paramount.
Applications of Federated Learning in Cybersecurity
Threat Detection and Anomaly Identification: Conventional threat detection methods frequently fall short in identifying and responding to the rapidly shifting tactics used by cyber attackers. FL enables real-time monitoring and collective analysis by pooling insights from diverse sources without exposing sensitive data. This dynamic approach allows for faster and more accurate identification of anomalies and potential threats.
Malware Detection and Classification: Malware constantly adapts to evade detection. FL brings a collective perspective to this challenge, allowing different organisations to contribute to robust malware classifiers without revealing specific datasets. The result is a more resilient defense against the ever-changing landscape of cyber threats.
Predictive Analysis for Cyber Attacks: FL enhances predictive models by collaboratively analyzing historical data from various entities. This proactive stance allows organisations to foresee potential attack vectors and adapt their defences accordingly, thwarting cyber threats before they strike.
Collaborative Defence Strategies: FL's collaborative nature enables organisations, even competitors, to share threat intelligence without exposing critical information. By working together, organisations can build a more resilient defence network, strengthening their overall cybersecurity framework against shared threats.
Privacy-Preserving Intrusion Detection: By keeping sensitive data local and sharing only anonymised insights, FL allows organisations to collectively identify new patterns of unauthorised access without compromising user confidentiality. This balance between privacy and security is crucial in today's data-sensitive environment.
Advantages of Federated Learning in Cybersecurity
Data Privacy and Compliance: FL ensures that sensitive data remains within its originating location, aligning with stringent data protection regulations such as GDPR and CCPA. This adherence to data sovereignty laws is crucial for organisations operating across multiple jurisdictions.
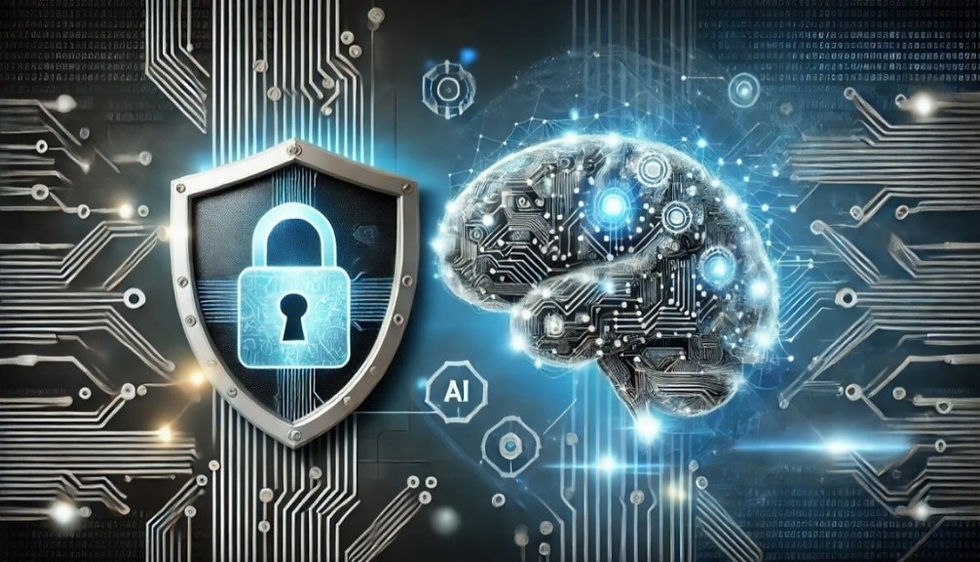
Enhanced Model Robustness: Training on diverse datasets from various sources makes models more adaptable and resilient. This diversity reduces the risk of biases and improves the model's ability to generalize across different scenarios.
Reduced Risk of Data Breaches: Since data is not transferred to a central server, the risk of data breaches during transmission is significantly reduced. This decentralised approach minimises potential vulnerabilities associated with data aggregation.
Scalability and Efficiency: FL allows for scalable model training across numerous devices or organisations without the need for centralised data storage. This scalability is particularly beneficial for large-scale cybersecurity applications.
Challenges and Considerations
While federated cybersecurity offers significant advantages, it is not without hurdles-
Computational Overhead: Encryption and decentralized processing require more resources than traditional methods.
Standardisation Issues: Lack of universal protocols can hinder interoperability between different federated systems.
Trust & Adoption Barriers: Some organisations remain sceptical about indirect data sharing and prefer traditional methods.
Latency in Threat Response: Federated models may introduce delays compared to real-time centralised threat feeds.
Data Quality and Consistency: Ensuring the quality and consistency of data across different clients is challenging. Variations in data formats, pre-processing methods, and quality can affect the performance of the global model.
Security of Model Updates: While FL protects raw data, the shared model updates can still be vulnerable to attacks. Protecting model updates requires advanced methods like secure aggregation and differential privacy to ensure sensitive information remains confidential.
Malicious Participants: Detecting and mitigating the impact of malicious participants who may submit poisoned data or model updates is a significant concern. Implementing robust validation and anomaly detection mechanisms is crucial.
Resource Constraints: FL can be computationally intensive, requiring significant processing power and bandwidth. Organisations must ensure they have the necessary infrastructure to support FL operations.
Real-World Implementations
Financial Sector: Fighting Fraud Without Sharing Customer Data - Banks and fintech firms use federated learning to detect fraudulent transactions without exposing customer records. For example, SWIFT’s Customer Security Programme (CSP) encourages collaborative defence without direct data sharing. JPMorgan Chase has explored federated AI to enhance anti-money laundering (AML) efforts.
Healthcare: Secure Medical Threat Intelligence Hospitals and research institutions collaborate on cyber-physical threats (like ransomware targeting medical devices) without violating patient confidentiality.

Critical Infrastructure: Protecting National Assets Government agencies and energy providers use federated models to defend against state-sponsored cyberattacks while keeping operational data confidential.
Enterprise Security: Cross-Company Threat Hunting Companies like IBM Security and Palo Alto Networks integrate federated techniques into their threat intelligence platforms to help clients share insights safely.
Future Outlook
As cyber threats continue to evolve, the adoption of federated learning in cybersecurity is expected to grow. Ongoing research aims to address current challenges, enhance the robustness of FL systems, and develop standardised protocols for broader implementation. Organizations looking to bolster their cybersecurity posture should consider integrating FL into their strategies, balancing the need for collaboration with the imperative of data privacy. As cyber threats evolve, federated cybersecurity will play an increasingly vital role in global cyber defence strategies. Emerging trends include-
Blockchain-based threat sharing: for tamper-proof collaboration.
AI-driven autonomous federated systems: that dynamically adapt to new threats.
Government-backed federated initiatives: such as the EU’s NIS2 Directive, promote cross-border cyber collaboration.
Conclusion
Federated cybersecurity represents a paradigm shift in how organisations defend against cyber threats. By enabling secure, privacy-preserving collaboration, it addresses critical challenges in threat intelligence sharing while complying with stringent data protection laws. As more industries adopt this model, we can expect a more resilient, interconnected cyber defence ecosystem—one where organisations work together without sacrificing data privacy.
Citations/References
Enterprise Security Solutions | IBM. (n.d.). https://www.ibm.com/security
NIS2 Directive: new rules on cybersecurity of network and information systems. (n.d.). Shaping Europe’s Digital Future. https://digital-strategy.ec.europa.eu/en/policies/nis2-directive
Customer Security Programme (CSP) | SWIFT. (n.d.). Swift. https://www.swift.com/myswift/customer-security-programme-csp
Wikipedia contributors. (2025, March 9). Federated learning. Wikipedia. https://en.wikipedia.org/wiki/Federated_learning
How is federated learning applied in security analytics? (n.d.). https://milvus.io/ai-quick-reference/how-is-federated-learning-applied-in-security-analytics
Manzoor, H. U., Shabbir, A., Chen, A., Flynn, D., & Zoha, A. (2024). A survey of security strategies in Federated Learning: Defending models, data, and Privacy. Future Internet, 16(10), 374. https://doi.org/10.3390/fi16100374
Secure AI. (2024, November 22). Harnessing the power of federated learning for enhanced cybersecurity. Medium. https://medium.com/%40secureai/harnessing-the-power-of-federated-learning-for-enhanced-cybersecurity-b42cb8ab3ac9
Pal, S. (2024, November 23). Applications of Federated Learning in Cyber-Security & Threat Intelligence | Medium. Medium. https://techunits.com/applications-of-federated-learning-in-cybersecurity-d599e33a3fe1
Liu, P., Xu, X., & Wang, W. (2022). Threats, attacks and defences to federated learning: issues, taxonomy and perspectives. Cybersecurity, 5(1). https://doi.org/10.1186/s42400-021-00105-6
Image Citations
Adams, D. (2024, August 15). Federal cybersecurity efforts seek to improve the protection of health data. Journal of AHIMA. https://journal.ahima.org/page/federal-cybersecurity-efforts-seek-to-improve-protection-of-health-data
Mark. (2024, May 28). Federated Learning: Collaborative AI Training without Sharing Raw Data. Zipfian Academy. https://www.zipfianacademy.com/federated-learning/
The Intersection of AI and Cybersecurity: A Double-Edged Sword | LinkedIn. (2024, September 13). https://www.linkedin.com/pulse/intersection-ai-cybersecurity-double-edged-sword-kelly-hammons-mdeac/
Hill, M. (2023, November 4). Exploring the intricacies of DMZ cybersecurity - CyberExperts.com. CyberExperts.com. https://cyberexperts.com/dmz-cyber-security/
Comments