The Convergence of Biotech and AI: Revolutionizing Drug Discovery and Accelerating Personalized Medicine
- Arpita (BISWAS) MAJUMDAR
- 5 days ago
- 9 min read
ARPITA (BISWAS) MAJUMDER | DATE: FEBRUARY 26, 2025
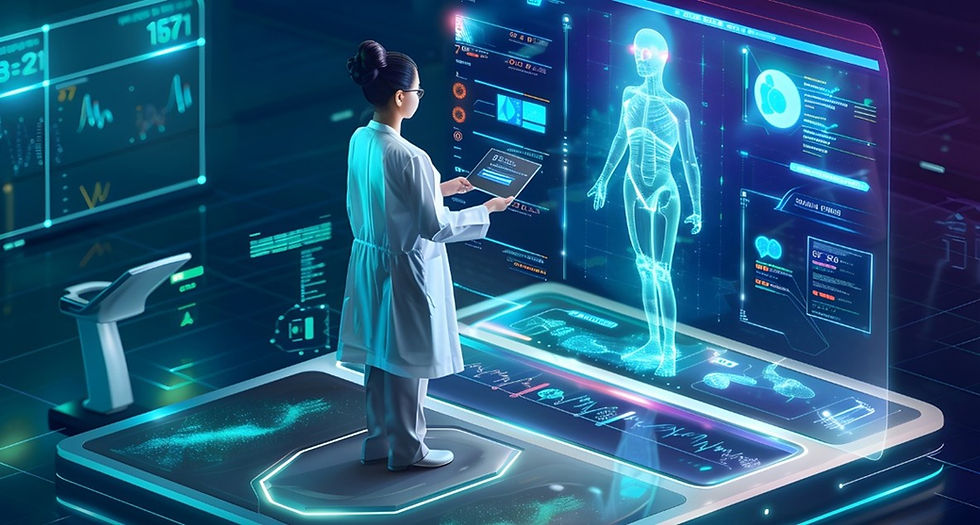
Introduction
Biotechnology and artificial intelligence (AI) have long operated as distinct pillars in modern healthcare—biotech, with its roots in harnessing biological systems to create therapies, and AI, a field that uses complex algorithms to analyze vast amounts of data. Today, however, these powerful disciplines are converging, creating a transformative synergy that is reshaping drug discovery and personalized medicine.
Historically, drug development has been an arduous, time-intensive process. Traditional methods rely heavily on laboratory experiments, trial-and-error approaches, and lengthy clinical trials, often taking over a decade and billions of dollars to bring a single new drug to market. Meanwhile, AI has been making strides in industries as diverse as finance, logistics, and technology by uncovering hidden patterns and making predictive analyses from large datasets. When these two worlds merge, the potential benefits are extraordinary.
AI-driven models can now rapidly analyse genetic, proteomic, and chemical data to identify novel therapeutic targets and predict the interactions between molecules and biological systems. This capability not only speeds up the identification of promising drug candidates but also enhances their design by suggesting modifications that improve efficacy and safety. For example, cutting-edge platforms can simulate the complex three-dimensional structures of proteins—once a painstaking process—thus providing crucial insights into how drugs might interact with their targets.
Beyond speeding up the discovery phase, the integration of AI into biotechnology is propelling the field toward personalized medicine. Instead of relying on the traditional “one-size-fits-all” approach, AI enables the tailoring of treatments to the individual by incorporating data from genomics, patient history, and real-time health monitoring. This means that therapies can be designed not only to combat diseases more effectively but also to match the unique biological makeup of each patient, ultimately leading to better clinical outcomes and fewer adverse effects.

In essence, the convergence of biotech and AI is forging a new paradigm—one where the complex challenges of drug discovery are met with unprecedented computational power, and where the promise of personalized, precision medicine becomes increasingly attainable. This article delves into how this integration is revolutionizing the way we discover, develop, and deliver therapies, setting the stage for a future where innovative treatments are both faster to develop and more finely tuned to individual patient needs.
A New Era in Drug Discovery
Traditional Challenges: For decades, drug discovery has been a laborious, expensive, and time-consuming process. Traditional methods rely on high-throughput screening, extensive laboratory testing, and sequential clinical trials that often take 10 to 15 years and cost billions of dollars. High failure rates in late-stage clinical trials further add to the financial risk. The inherent complexity of biological systems, combined with the trial-and-error approach in discovering active compounds, has led to an urgent need for more efficient and predictive methodologies.
The Emergence of AI: Enter artificial intelligence. With its ability to analyse and interpret vast quantities of data, AI has emerged as a powerful tool to address these challenges. Machine learning algorithms and deep neural networks can now rapidly sift through enormous chemical libraries, genomic databases, and clinical records to identify potential drug candidates with unprecedented speed and accuracy. By simulating molecular interactions and predicting biological activities, AI-driven platforms are enabling researchers to narrow down promising compounds far earlier in the drug development process.
For example, DeepMind’s AlphaFold has revolutionized our understanding of protein structures by predicting their 3D configurations with near-experimental accuracy in a matter of hours—a task that once took years of laboratory work. This breakthrough not only informs the design of more effective therapeutics, but also accelerates the drug discovery process by revealing critical insights into how proteins interact with potential drugs. DeepMind AlphaFold.
AI-Enhanced Virtual Screening and De Novo Design: AI’s role in virtual screening has been transformative. Instead of manually testing thousands of compounds, researchers can now use machine learning models to simulate and predict which molecules are most likely to exhibit desired therapeutic properties. Additionally, generative AI models are being employed for de novo drug design. These models generate novel chemical structures that are optimized for target specificity, efficacy, and safety. This not only opens up vast new chemical spaces but also allows for the design of drugs tailored to treat diseases that were previously deemed “undruggable.”
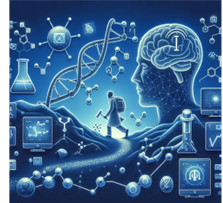
Accelerating Personalized Medicine
Beyond “One-Size-Fits-All”: Traditional drug development has largely followed a one-size-fits-all paradigm, where medications are developed for the “average” patient. However, the realization that no two patients are exactly alike has fueled the shift toward personalized medicine. By integrating individual genetic, proteomic, and clinical data, AI enables the development of treatments that are tailored to the unique biological makeup of each patient.
Integrating Big Data for Individualized Therapies: The convergence of AI and biotechnology has made it possible to integrate diverse data types—from electronic health records and wearable device metrics to genomic and proteomic information—into comprehensive predictive models. These models help in:
Identifying Biomarkers: Advanced algorithms can uncover genetic and protein markers that predict how a patient will respond to a particular drug, leading to better patient stratification.
Predicting Drug Response: AI can forecast therapeutic outcomes and potential adverse effects, enabling clinicians to choose the most effective treatment regimen.
Dynamic Treatment Adjustment: Real-time data from ongoing treatment can be used to adjust dosages and modify therapeutic strategies, ensuring that interventions remain optimal as the patient’s condition evolves.
Companies such as Insilico Medicine and Owkin are at the forefront of this revolution. Insilico Medicine uses AI to identify novel drug targets and design new molecules, while Owkin employs federated learning to harness data from multiple institutions without compromising patient privacy Insilico Medicine • Owkin.
Digital Twins and Predictive Analytics: One of the most futuristic and promising applications of AI in personalized medicine is the development of digital twins—virtual replicas of individual patients that simulate their unique biological processes. Digital twins allow clinicians to test different therapeutic strategies in a simulated environment, significantly reducing the risk of adverse reactions and optimizing treatment plans. Predictive analytics, powered by AI, further refine this process by identifying which patients are most likely to benefit from a particular therapy, thus streamlining clinical trials and enhancing patient outcomes.
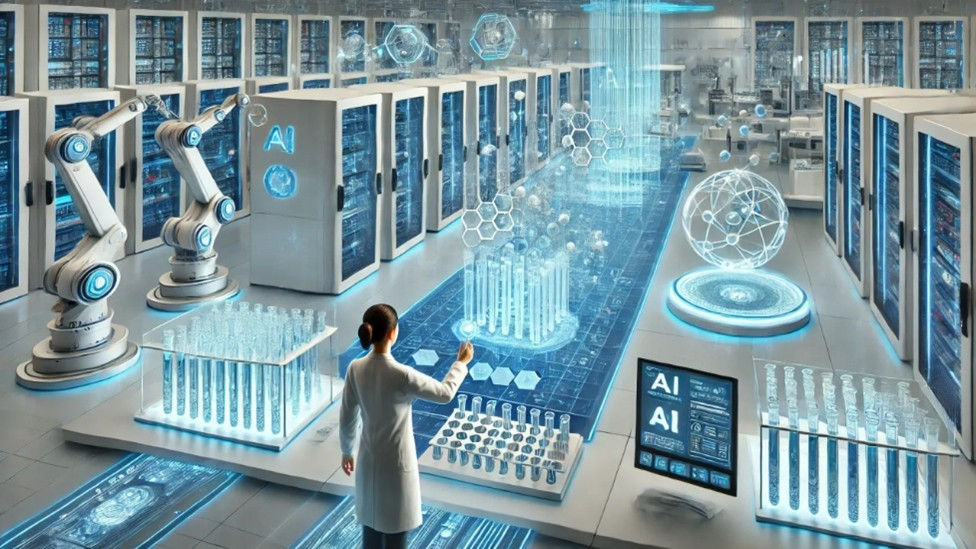
Case Studies: Pioneering Innovations in AI-Driven Biotech
DeepMind’s AlphaFold: DeepMind’s AlphaFold is a landmark achievement that has fundamentally changed protein structure prediction. By using deep learning to model the three-dimensional structures of proteins, AlphaFold has provided researchers with critical insights into molecular interactions that inform drug design. Its rapid predictions have accelerated research in fields ranging from oncology to neurodegenerative diseases. DeepMind AlphaFold.
Insilico Medicine’s AI Platform: Founded in 2014, Insilico Medicine applies deep learning to drug discovery, integrating genomics, big data, and chemical biology to identify novel drug targets and design new therapeutics. The company has reached significant milestones, including initiating mid-stage clinical trials for an AI-designed drug. Insilico’s platform exemplifies how AI can shorten development timelines and improve success rates by optimizing drug properties early in the discovery process Insilico Medicine.
Owkin’s Federated Learning Approach: Owkin, a French biotech firm, has pioneered the use of federated learning in healthcare. This method allows multiple institutions to collaboratively train AI models without exchanging sensitive patient data, thereby preserving privacy while harnessing vast datasets. Owkin’s diagnostic tool, MSIntuit CRC, uses this technology to screen for microsatellite instability in colorectal cancer, enhancing both diagnostic accuracy and patient-specific treatment strategies. Owkin.

Isomorphic Labs: DeepMind’s Drug Discovery Spin-Off: Isomorphic Labs, a spin-off from DeepMind, leverages the insights gained from AlphaFold to design novel therapeutics. By integrating AI with traditional wet lab research, Isomorphic Labs is working in collaboration with major pharmaceutical companies like Novartis and Eli Lilly to bring new, effective drugs to market faster. Their work underscores the critical role of AI in not only understanding biological systems but also in designing molecules with the desired therapeutic effects Isomorphic Labs.
Challenges and Future Perspectives
Data Quality and Integration: The success of AI-driven drug discovery hinges on the quality and diversity of input data. Incomplete or biased datasets can lead to inaccurate predictions and limit the effectiveness of AI models. Efforts to integrate disparate data sources—from genomics and proteomics to clinical and lifestyle information—are essential to creating robust, generalizable models.
Regulatory and Ethical Considerations: As AI-driven methodologies reshape drug discovery and personalized medicine, regulatory frameworks must evolve to keep pace. Ensuring the safety, efficacy, and transparency of AI-generated insights is a major challenge. Regulatory bodies worldwide are working to establish guidelines that balance innovation with patient safety while addressing concerns related to data privacy and algorithmic bias.
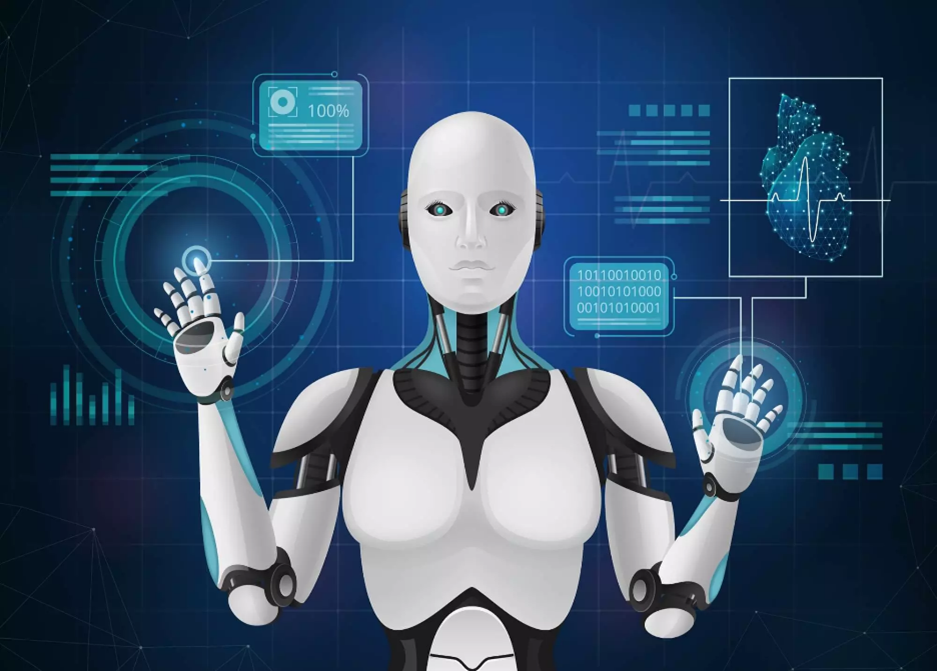
Bridging the Gap Between AI and the Laboratory: While AI has demonstrated remarkable potential in virtual environments, translating these predictions into successful clinical outcomes remains challenging. Bridging the gap between computational predictions and wet lab validations requires ongoing collaboration between data scientists, clinicians, and biologists. Initiatives that foster such cross-disciplinary cooperation are crucial for the continued success of AI in drug development.
The Future of AI in Healthcare: Looking ahead, the integration of AI into biotech is poised to continue its transformative trajectory. With advancements in reinforcement learning, transfer learning, and the development of foundation models, AI will become increasingly adept at predicting complex biological interactions and tailoring therapies to individual patients. The future may see the emergence of fully digital drug discovery pipelines and personalized treatment regimens that evolve in real time, fundamentally altering the healthcare landscape.
Conclusion
The convergence of biotechnology and artificial intelligence is ushering in a new era in healthcare—one where the lengthy, costly process of drug discovery is dramatically accelerated, and therapies are increasingly personalized to meet individual patient needs. By harnessing AI’s computational power to analyse vast datasets, predict molecular behaviour, and simulate biological processes, researchers are not only speeding up the discovery and development of new drugs but also crafting treatments that are more effective and safer.
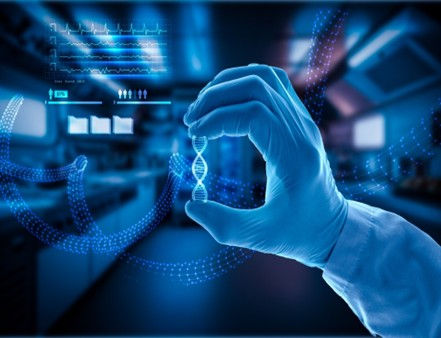
As the integration of AI into biotech continues to mature, we can expect a future where innovative treatments are developed more rapidly, clinical trials become more efficient, and the overall cost of healthcare is reduced. This revolution promises not only to transform the pharmaceutical industry but also to fundamentally improve patient outcomes, offering hope for better, more precise, and personalized care.
Citations/References
AI meets real-world patients. (2024). Nature Biotechnology, 42(10), 1475. https://doi.org/10.1038/s41587-024-02455-1
Morgan Stanley. (n.d.). How AI could speed drug discovery | Morgan Stanley. https://www.morganstanley.com/ideas/ai-drug-discovery
Dealmakers, B. (2024). Generative AI platforms drive drug discovery dealmaking. Biopharma Dealmakers. https://doi.org/10.1038/d43747-024-00084-w
Albert, H., & Albert, H. (2023, August 21). These Six Biotechs are Winning the Race to Get AI-Designed Drugs to the Clinic. Inside Precision Medicine. https://www.insideprecisionmedicine.com/news-and-features/these-six-biotechs-are-winning-the-race-to-get-ai-designed-drugs-to-the-clinic/
Serrano, D. R., Luciano, F. C., Anaya, B. J., Ongoren, B., Kara, A., Molina, G., Ramirez, B. I., Sánchez-Guirales, S. A., Simon, J. A., Tomietto, G., Rapti, C., Ruiz, H. K., Rawat, S., Kumar, D., & Lalatsa, A. (2024). Artificial intelligence (AI) applications in drug discovery and drug delivery: Revolutionizing personalized medicine. Pharmaceutics, 16(10), 1328. https://doi.org/10.3390/pharmaceutics16101328
Vamathevan, J., Clark, D., Czodrowski, P., Dunham, I., Ferran, E., Lee, G., Li, B., Madabhushi, A., Shah, P., Spitzer, M., & Zhao, S. (2019). Applications of machine learning in drug discovery and development. Nature Reviews Drug Discovery, 18(6), 463–477. https://doi.org/10.1038/s41573-019-0024-5
Jumper, J., Evans, R., Pritzel, A., Green, T., Figurnov, M., Ronneberger, O., Tunyasuvunakool, K., Bates, R., Žídek, A., Potapenko, A., Bridgland, A., Meyer, C., Kohl, S. a. A., Ballard, A. J., Cowie, A., Romera-Paredes, B., Nikolov, S., Jain, R., Adler, J., . . . Hassabis, D. (2021). Highly accurate protein structure prediction with AlphaFold. Nature, 596(7873), 583–589. https://doi.org/10.1038/s41586-021-03819-2
Topol, E. J. (2018). High-performance medicine: the convergence of human and artificial intelligence. Nature Medicine, 25(1), 44–56. https://doi.org/10.1038/s41591-018-0300-7
Wornow, M., Xu, Y., Thapa, R., Patel, B., Steinberg, E., Fleming, S., Pfeffer, M. A., Fries, J., & Shah, N. H. (2023). The shaky foundations of large language models and foundation models for electronic health records. Npj Digital Medicine, 6(1). https://doi.org/10.1038/s41746-023-00879-8
ElZarrad, K., et al. (2023). Discussion paper and request for feedback using artificial intelligence & machine learning in the development of drug & biological products. In Food and Drug Administration (p. 1). https://www.fda.gov/media/167973/download
Image Citations
Oswal, N. M. (2024, November 19). Part 10: How GenAI is Revolutionizing Healthcare: From Drug Discovery to Digital Health - Kae Capital. Kae Capital. https://kae-capital.com/blogs/part-10-how-genai-is-revolutionizing-healthcare-from-drug-discovery-to-digital-health/
(29) Revolutionizing Drug Discovery: AI in Regenerative Medicine | LinkedIn. (2024, July 25). https://www.linkedin.com/pulse/revolutionizing-drug-discovery-ai-regenerative-medicine-sanket-gadhe-91thf/
Ferguson, M. (2025, February 13). Ex-DeepMind scientist embarks on AI-Driven drug discovery adventure! Ex-DeepMind Scientist Embarks on AI-Driven Drug Discovery Adventure! https://opentools.ai/news/ex-deepmind-scientist-embarks-on-ai-driven-drug-discovery-adventure
(29) Accelerating Drug Discovery with AI: A Next-Generation Platform for Efficient Candidate Identification and Lead Optimization | LinkedIn. (2024, October 29). https://www.linkedin.com/pulse/accelerating-drug-discovery-ai-next-generation-lead-ramachandran-dqn0c/
Edwards, J. (2024, July 26). Revolutionising Drug Discovery: AI Advances with Insilico Medicine's PandaOmics Box | Pharma IQ. Pharma IQ. https://www.pharma-iq.com/pre-clinical-discovery-and-development/articles/revolutionising-drug-discovery-ai-advances-with-insilico-medicines-pandaomics-box
Koniukh, A., & Koniukh, A. (2023, August 17). Emerging technologies in biotech: Exploring AI and machine learning. Nanobot. https://nanobotmedical.com/blog/emerging-technologies-in-biotech-exploring-ai-and-machine-learning/
(29) The Impact of Artificial Intelligence on pharmaceutical Innovation | LinkedIn. (2024, April 3). https://www.linkedin.com/pulse/impact-artificial-intelligence-pharmaceutical-barrag%C3%A1n-bioconsulting-nkgbf/
About the Author
Arpita (Biswas) Majumder is a key member of the CEO's Office at QBA USA, the parent company of AmeriSOURCE, where she also contributes to the digital marketing team. With a master’s degree in environmental science, she brings valuable insights into a wide range of cutting-edge technological areas and enjoys writing blog posts and whitepapers. Recognized for her tireless commitment, Arpita consistently delivers exceptional support to the CEO and to team members.
Comments