Data Science: Charting the Unexplored Frontier of Tomorrow
- Probal DasGupta
- 5 days ago
- 12 min read
PROBAL DASGUPTA | DATE: MARCH 19, 2024
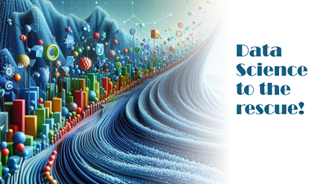
In the age of digital transformation, data science has emerged as the driving force reshaping industries, businesses, and even how we perceive daily life. As we unravel the intricate tapestry of data, we see a narrative that interweaves everything from technological advancement to human insight, promising not only efficiency and innovation but a fundamentally different world. The journey of data science is only beginning, and the scope of its potential is as vast as our imaginations.
The Dawn of Data Science: An Era Defined by Information
The past few decades have seen the rapid accumulation of data, transforming it into a resource as valuable as any natural commodity. From social media interactions to financial transactions and health records, we are generating data at an unprecedented rate. This exponential growth has sparked a revolution—one that is reshaping industries, driving business strategies, and even influencing government policies. Organizations across the world are no longer just tracking every facet of operations; they are actively seeking ways to extract meaningful insights from this vast ocean of information.
In this new era, data science has emerged as an indispensable tool. It's not merely about gathering data but about transforming raw numbers into compelling narratives and actionable strategies. Data scientists are the modern-day alchemists, taking unrefined data and turning it into gold—whether it’s predicting customer behavior, enhancing operational efficiencies, or even uncovering new market opportunities. But data science is as much about the numbers and algorithms as it is about understanding the human behavior behind them. How people interact with technology, what they value, and how they make decisions are crucial insights that can inform business strategies, social policies, and technological development.

Yet, as powerful as data science is, it comes with its own set of ethical challenges. With great power comes great responsibility. The manipulation of data, if done without transparency and consideration, can lead to unintended consequences. Data breaches, algorithmic biases, and privacy violations have become major concerns in the digital age. As such, the field of data science isn’t only about innovation—it’s about the careful navigation of the ethical, legal, and moral boundaries that come with the power of information. Today’s data scientists must balance the drive for progress with an unwavering commitment to responsible data stewardship, ensuring that the benefits of data-driven decisions reach all corners of society without compromising individual rights or societal trust.
In the coming years, the role of data science will continue to grow and evolve. As artificial intelligence (AI), machine learning (ML), and big data technologies advance, the potential to unlock deeper, more profound insights will expand. At the same time, the ability to influence and predict human behavior, as well as automate decisions, will prompt more complex questions about the future of work, privacy, and fairness in our increasingly data-driven world.
Foundations of a Data-Driven World
The foundations of a data-driven world rest on innovations in machine learning, artificial intelligence, and predictive analytics, which together enable powerful insights from vast datasets. Algorithms play a critical role by analyzing complex data patterns across sectors:
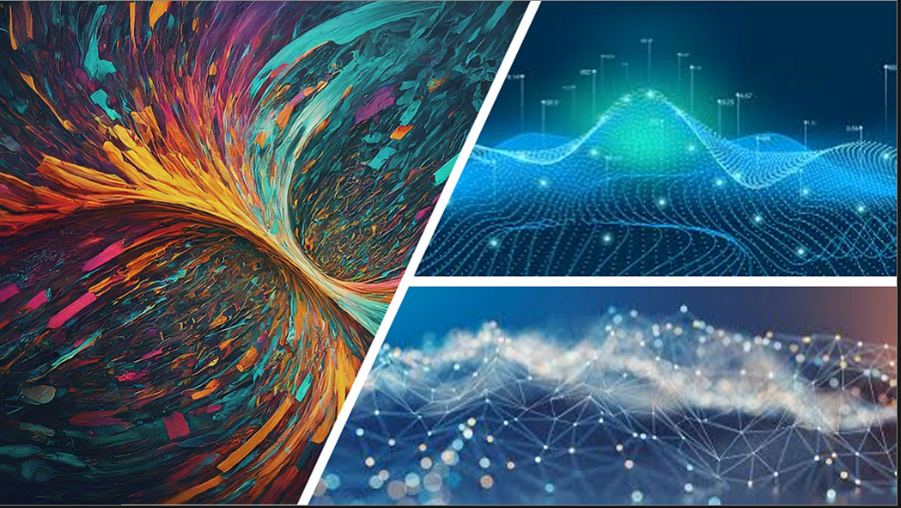
In Healthcare, for instance, AI-driven models assist in diagnosing diseases by recognizing early symptoms from patient data.
In Finance, predictive analytics assess credit risk and detect fraud by identifying unusual transaction patterns.
However, human expertise remains essential in refining these algorithms to prevent biases and ensure ethical, actionable outcomes.
Data scientists, engineers, and industry specialists work collaboratively to apply these tools effectively, tailoring solutions to real-world contexts such as optimizing supply chains in manufacturing, enhancing personalized customer experiences in retail, and even aiding in urban planning for smarter cities.
This convergence of technology and human insight is not just advancing individual industries but is shaping a new workforce equipped to drive informed, data-centered decision-making across all areas of modern society.
Data Science Across Industries
Data science is far from confined to a single domain. It has infiltrated nearly every sector, shaping the way organizations operate, innovate, and interact with their customers. Its applications are vast, and the impact it has across industries is profound. What was once considered a niche field has now become a driving force for transformation, fuelling innovation and efficiency.

Healthcare Sector
In healthcare, predictive analytics powered by data science has revolutionized the way diseases are diagnosed and treated. Beyond forecasting disease outbreaks, data science is playing a critical role in personalized medicine, where algorithms analyze genetic data, patient histories, and environmental factors to tailor treatments to individual needs. The field of genomics, in particular, is benefitting from data science, as researchers sift through vast datasets to identify potential genetic markers for diseases, creating a new era of preventative medicine. AI-powered diagnostic tools are also assisting doctors by providing more accurate readings from medical imaging, reducing human error, and speeding up diagnoses.
Finance Sector
In finance, the integration of data science has fundamentally transformed everything from fraud detection to investment strategies. Machine learning models analyze transaction patterns in real-time to identify fraudulent activity with remarkable precision, helping banks and financial institutions mitigate risk. Data science also aids in personalizing financial services, from offering customized investment portfolios to creating algorithms that suggest savings plans based on a person’s financial behavior. Risk management is another area that has benefited greatly. Predictive models assess market fluctuations, helping firms hedge against potential risks and make informed decisions about investments and assets. These advancements allow both consumers and organizations to benefit from more tailored and secure financial solutions.
Transportation Sector
The transportation sector has also seen tremendous benefits from data science, particularly in the realms of logistics, maintenance, and route optimization. Companies like Uber, Lyft, and autonomous vehicle manufacturers use real-time data to improve routing algorithms, ensuring that passengers reach their destinations quickly and drivers take the most efficient paths. In aviation, data science is instrumental in managing air traffic and improving fuel efficiency. Moreover, predictive analytics enables companies to foresee maintenance needs before breakdowns occur, minimizing downtime and repair costs. As the demand for sustainability increases, data science also helps optimize transportation systems to reduce carbon emissions and improve energy efficiency.
Retail Industry
The retail industry has been significantly transformed by the data-driven insights that data science provides. Retailers use data science to track and predict consumer behavior, which allows for personalized shopping experiences. By analyzing purchasing habits, retailers can recommend products in real-time or create targeted advertising campaigns that resonate with individual consumers. Pricing strategies are also fine-tuned with dynamic pricing models powered by data science, where prices are adjusted in real-time based on demand, competitor pricing, and other external factors. In e-commerce, recommendation algorithms — like those used by Amazon or Netflix — not only increase customer satisfaction but also boost sales, as they make it easier for consumers to discover products that fit their preferences.
Manufacturing
In manufacturing, data science enhances operational efficiency by analyzing data from production lines in real time. This allows companies to optimize processes, reduce waste, and improve product quality. Predictive maintenance, similar to transportation, plays a crucial role in identifying equipment failures before they happen, ensuring minimal disruption to production. In supply chain management, data science enables the monitoring and optimization of inventory, logistics, and demand forecasting, which can significantly reduce costs and improve overall efficiency.
Education Industry
Education is another industry increasingly benefitting from data science. With the vast amount of data generated by learning management systems (LMS), educators can now analyze student performance and personalize learning experiences. Adaptive learning platforms use AI to tailor lessons to individual students' needs, helping them learn at their own pace and improve retention. In higher education, data science is also applied in student admissions, alumni tracking, and optimizing curricula to meet the evolving demands of the workforce.
In energy sectors, data science is helping companies optimize the use of renewable resources, track energy consumption patterns, and predict future energy needs. Smart grids use real-time data to monitor and manage the distribution of electricity, making the energy system more resilient, efficient, and responsive. By applying data science, energy firms can also forecast potential outages or system failures, enabling quicker responses and reduced downtime.
Government Sector
Even the government sector is tapping into data science to improve public services and governance. From urban planning and traffic management to predictive policing and disaster response, data science is enhancing the efficiency of government operations. In some cases, predictive models help governments prepare for natural disasters by analyzing weather patterns, geological data, and infrastructure readiness. In law enforcement, data analysis is used to predict crime hotspots, optimize patrol routes, and allocate resources more effectively.
As data science continues to evolve, its reach will only expand further, driving innovation, efficiency, and opportunity across every industry. What’s clear is that data science is not just a tool for optimization, but a transformative force that can unlock entirely new ways of operating, solving problems, and delivering value.
The Role of Artificial Intelligence and Machine Learning
Artificial Intelligence (AI) and Machine Learning (ML) are at the heart of the data science revolution, transforming the landscape of business, healthcare, technology, and beyond. These technologies have taken data science from basic analytics to complex, real-time decision-making processes that can learn, adapt, and evolve without direct human intervention. While data science involves deriving insights from data, AI and ML bring an unprecedented ability to automate processes, enhance predictions, and improve efficiency on a massive scale.
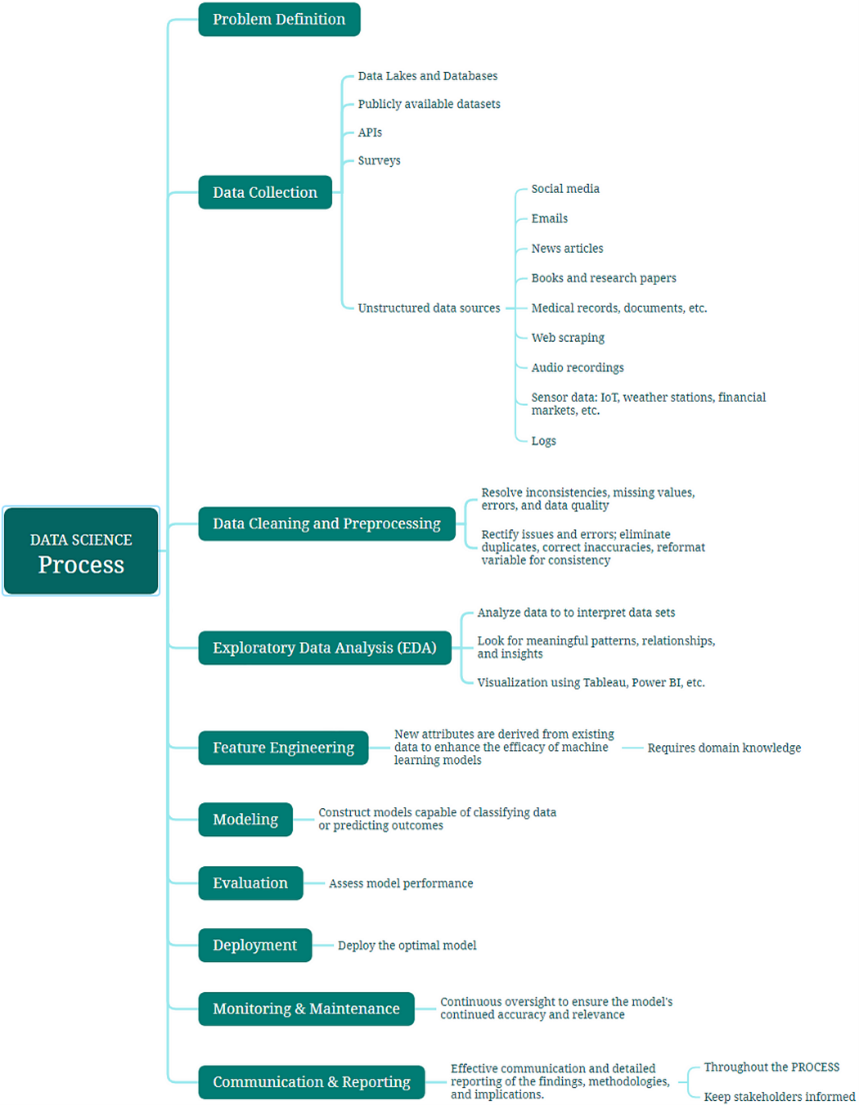
Machine Learning: Learning from Data Patterns
At its core, Machine Learning, a subset of AI, allows systems to "learn" from data patterns. These algorithms are trained on vast datasets, enabling them to identify patterns, make predictions, and continuously refine their outputs based on new information. Unlike traditional systems, which require explicit instructions, ML models can improve autonomously as they process more data, creating a feedback loop that enhances performance over time. Whether it’s a recommendation engine predicting a user’s next purchase or a diagnostic algorithm identifying early signs of disease, ML algorithms can adapt to changing conditions and optimize their predictions with every new piece of data.
AI in Fraud Detection
AI’s impact is particularly noticeable in fraud detection, where it processes millions of transactions in real-time. Traditional methods, such as manual review or rule-based detection systems, are no longer sufficient in a world with vast volumes of data. AI algorithms, powered by machine learning, can sift through massive datasets and detect anomalies that would be impossible for human analysts to catch. By recognizing patterns of legitimate behavior, AI models can quickly flag outliers—potential frauds—and even stop fraudulent transactions within seconds, mitigating risks for financial institutions and customers alike.
AI and Machine Learning in Autonomous Vehicles
In autonomous vehicles, AI and machine learning have completely redefined the transportation landscape. Autonomous cars and drones navigate the world by processing vast amounts of spatial and environmental data in real-time. They use machine learning models to recognize road signs, pedestrians, obstacles, and even other vehicles, allowing them to make split-second decisions that are far more accurate than human drivers could ever achieve. Over time, these systems learn from each mile traveled, adapting to new driving conditions, detecting risks before they arise, and optimizing routes. The end result is not only a safer driving experience but also one that minimizes human error, traffic congestion, and carbon emissions.
AI and machine learning have permeated industries far beyond fraud detection and autonomous driving, pushing the boundaries of what's possible.
Transforming Healthcare with AI
In healthcare, AI models are used to analyze medical imaging with incredible precision, often detecting diseases like cancer or neurological disorders at their earliest stages, when they are most treatable. With the help of machine learning, AI can analyze patient data, medical histories, and genetic information to develop personalized treatment plans, predicting outcomes and recommending the most effective therapies based on individual needs. AI is also transforming drug discovery, enabling researchers to rapidly test new compounds and accelerate the process of bringing life-saving drugs to market.
AI and Machine Learning in Manufacturing
In manufacturing, AI and ML are central to the growing trend of "smart factories." By integrating IoT (Internet of Things) devices, machine learning models can predict equipment failures before they happen, optimizing maintenance schedules, and minimizing costly downtimes. AI-powered systems can monitor production lines in real-time, identifying inefficiencies or quality issues that may have been overlooked by human workers. By continuously learning from the production environment, these systems not only enhance operational efficiency but also improve the safety and quality of products, leading to reduced waste and increased profitability.
AI-Powered Innovation Across Sectors
One of the most transformative aspects of AI is its ability to drive innovation across virtually every sector. AI-powered Natural Language Processing (NLP) models, for example, have revolutionized customer service, enabling businesses to provide instant responses to customer queries through chatbots and virtual assistants. NLP technology has also been instrumental in automating content generation, making it easier for marketers to create personalized and relevant messages at scale. In retail, AI is driving personalized shopping experiences by analyzing customer data to recommend products in real-time. Personalized pricing models powered by AI adjust in response to consumer demand, inventory levels, and competitor pricing, helping retailers optimize their sales strategies and customer satisfaction.
AI in Finance
In the realm of finance, AI and ML are used to model stock prices, predict market trends, and even automate high-frequency trading strategies. Hedge funds and investment firms are increasingly turning to AI-powered algorithms to uncover hidden market patterns, allowing them to make faster and more accurate investment decisions than ever before. AI-driven models are also being employed to assess creditworthiness, with machine learning algorithms analyzing a wider range of data points—from social media activity to transaction history—giving lenders a more nuanced and comprehensive understanding of a borrower’s risk profile.
Perhaps most exciting of all is the ongoing potential for AI to learn and adapt in real-time.
Reinforcement Learning: The Future of AI
As the field of AI and machine learning continues to evolve, new models and technologies emerge, enabling even more advanced decision-making capabilities. For instance, reinforcement learning, a type of machine learning where systems learn by interacting with their environment and receiving feedback, is being explored for applications in robotics, gaming, and beyond. These systems learn through trial and error, optimizing their strategies and actions as they gain more experience, making them capable of handling highly dynamic and uncertain environments.
The Rapid Evolution of AI and Machine Learning
The speed at which AI and machine learning evolve is staggering, and as these technologies advance, the boundaries of what’s possible continue to expand. It’s clear that AI is not just a tool—it’s becoming the brain behind the scenes, driving decision-making across industries and enabling organizations to harness data like never before.
Challenges in Data Science: Navigating Complexities and Ethical Concerns
With the rise of data science, ethical concerns have come into sharper focus. Data privacy, algorithmic transparency, and fairness are no longer fringe concerns but central issues shaping public trust in technology. Companies face mounting pressure to ensure they respect individual privacy and avoid perpetuating biases in their algorithms. Additionally, there is the challenge of handling data responsibly, as regulatory frameworks around data protection grow stricter globally. In response, organizations are beginning to place ethics and accountability at the core of their data science strategies.
As data-driven decision-making continues to revolutionize industries, the demand for skilled data professionals has skyrocketed. However, despite the rapid growth of data science programs and initiatives, there remains a significant shortage of qualified talent capable of meeting the diverse needs of today's data-centric organizations. To address this growing gap, companies and educational institutions are exploring various strategies to build a robust workforce that can drive innovation and success in the data science space, the practices that needs to be follow are-
1. Evolving Education and Training Programs
2. Industry-Specific Expertise
3. Building Cross-Functional Teams
4. Upskilling and Reskilling Initiatives
5. The Role of Artificial Intelligence and Automation in Talent Development
6. Attracting and Retaining Talent
7. Government and Industry Initiatives
Bridging the Skills Gap: Building the Workforce of Tomorrow

Data science is no longer just a tool for making business decisions; it has become a transformative force reshaping virtually every aspect of modern life. With the exponential growth of data, advanced analytics, and machine learning algorithms, the possibilities for leveraging data science seem endless. As the field continues to evolve, its potential to tackle some of the world’s most pressing challenges grows larger, offering solutions to complex problems and creating opportunities for unprecedented advancements across industries.
The demand for skilled data professionals continues to surge, yet there is a notable shortage in qualified talent. While educational institutions rush to incorporate data science into their curricula, industry-specific expertise remains invaluable. Training programs, certifications, and even cross-functional team structures have become essential strategies for closing the skills gap. The rise of interdisciplinary teams, where data scientists work closely with professionals from finance, marketing, healthcare, and more, demonstrates a shift toward a collaborative approach in problem-solving.
Looking Forward: Data Science’s Limitless Future
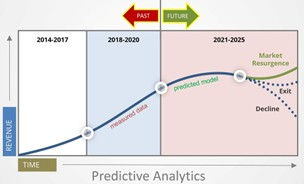
The impact of data science is unfolding with unprecedented scope and speed. As we stand on the brink of an era where algorithms could predict global economic shifts or prevent pandemics, the journey has only begun.
Researchers are paving the way for real-time decision-making in industries like finance, agriculture, and public safety. In education, data-driven insights are enabling tailored learning experiences that adapt to individual needs, while in environmental science, predictive models are helping to address climate challenges by forecasting natural disasters and monitoring biodiversity. They are exploring ways to leverage data science for more precise climate modeling, smarter cities, and even predicting human behavior with remarkable accuracy. Each new development promises to add another layer to this transformative science, bringing with it the hope of a better, more informed world.
Epilogue: A Journey of Endless Possibility

Data science stands as a transformative force, reshaping industries and impacting lives in ways we are only beginning to comprehend. Its potential stretches beyond today’s advancements, promising innovations that will redefine how we approach complex issues like healthcare accessibility, environmental sustainability, and global economic stability. As data science matures, ethical considerations and transparency will play pivotal roles, ensuring that insights are used responsibly to benefit society. The collective efforts of the data science community foster a spirit of openness and inclusivity, bringing together diverse perspectives to tackle the world’s most pressing challenges. In this shared journey, data science isn’t merely a tool—it's a catalyst for positive change, guiding humanity toward an era of unprecedented understanding and progress.
Conclusion: A Bright Future on the Horizon
As we look to the future, the data science journey will continue to unfold, bringing with it transformative opportunities and challenges. However, we can take comfort in knowing that, with the collaborative spirit that defines the global data science community, there is every reason to be optimistic. Together, we can turn the vast ocean of data into a powerful resource—a tool for innovation and a bright future for all.
Explanations:
A. The definition of "data" can vary, and sources might include or exclude certain data types in their calculations.
B. The Sun is 93 million miles from the Earth, while Proxima Centauri is 24,984 billion miles away. These astronomical distances give a scale to the vast amounts of data being discussed.
C. A shift is happening in data analytics, with the focus transitioning away from descriptive analytics. Studies from McKinsey and Forbes indicate that 60%-70% of data analysis activities were still descriptive as of 2023, but there is growing push toward predictive and prescriptive analytics.
留言