From Big Data to Smart Insights: The Role of Machine Learning in Analytics
- Jukta MAJUMDAR
- 5 days ago
- 3 min read
JUKTA MAJUMDAR | DATE: January 13, 2025

Introduction
In today's data-driven world, organizations are inundated with vast amounts of information, often referred to as "big data." However, raw data alone holds little value. The true power lies in extracting meaningful insights that can drive informed decision-making. This is where machine learning (ML) plays a crucial role, transforming traditional analytics into a more sophisticated and insightful process. This article explores how machine learning empowers analytics to uncover hidden patterns, predict future trends, and ultimately generate actionable intelligence.
The Evolution of Analytics

Traditional analytics methods often rely on descriptive statistics and historical data to understand past performance. While valuable, these methods lack the ability to predict future outcomes or uncover complex relationships within large datasets. Machine learning enhances analytics by automating the process of pattern discovery and prediction. By training algorithms on historical data, these models can learn underlying patterns and then apply that knowledge to new data, making predictions and generating insights that would be impossible to obtain through traditional methods.
Machine Learning Techniques in Analytics
Various machine learning techniques are employed in analytics to address different challenges:
Supervised Learning
The Supervised learning involves training models on labeled data, where the desired output is known. Examples include classification (categorizing data into predefined classes) and regression (predicting continuous values). In analytics, supervised learning can be used for tasks like customer churn prediction, fraud detection, and sales forecasting.
Unsupervised Learning
This involves training models on unlabeled data, where the desired output is not known. The goal is to discover hidden patterns and structures within the data. Examples include clustering (grouping similar data points together) and dimensionality reduction (reducing the number of variables while preserving important information). In analytics, unsupervised learning can be used for customer segmentation, anomaly detection, and market basket analysis.
Reinforcement Learning
This involves training an agent to interact with an environment and learn through trial and error. While less common in traditional analytics, reinforcement learning is gaining traction in areas like dynamic pricing and personalized recommendations.
Applications in Various Domains
The application of machine learning in analytics spans across various industries:
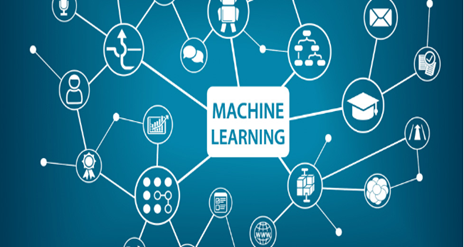
Marketing and Sales
Machine learning can be used to personalize marketing campaigns, optimize pricing strategies, and predict customer behavior.
Finance
Machine learning can be used for fraud detection, risk assessment, and algorithmic trading.
Healthcare
Machine learning can be used for disease diagnosis, personalized treatment plans, and drug discovery.
Manufacturing
Machine learning can be used for predictive maintenance, quality control, and supply chain optimization.
From Data to Actionable Insights
Machine learning transforms raw data into actionable insights by:
Automating Pattern Discovery
ML algorithms can automatically identify complex patterns and relationships within large datasets, saving analysts significant time and effort.
Predicting Future Trends
By learning from historical data, ML models can forecast future trends and outcomes, enabling proactive decision-making.
Generating Personalized Recommendations
ML can be used to create personalized recommendations for products, services, and content, enhancing customer experience and driving engagement.
Conclusion
Machine learning has become an indispensable tool in the field of analytics, enabling organizations to extract valuable insights from ever-growing volumes of data. By automating pattern discovery, predicting future trends, and generating personalized recommendations, machine learning empowers businesses to make smarter decisions, optimize operations, and gain a competitive edge. As data continues to grow in volume and complexity, the role of machine learning in analytics will only become more critical in driving innovation and success.
Sources
Mostert, B. (2023, January 31). What is machine learning for analytics? Oracle. https://www.oracle.com/business-analytics/what-is-machine-learning-for-analytics/
Chen, M. (2024, October 22). Machine learning and analytics: An expert guide. Oracle. https://www.oracle.com/business-analytics/machine-learning/
ABAC® (n.d.). The future of data analytics: AI and machine learning trends. https://iabac.org/blog/the-future-of-data-analytics-ai-and-machine-learning-trends
Image citation
WEKA. (2024, July 31). Big Data & Machine Learning (How do they relate?) - WEKA. https://www.weka.io/learn/glossary/ai-ml/big-data-machine-learning/
(29) The Critical Role of Artificial intelligence / Machine Learning in big data a nalytics | LinkedIn. (2023, September 27). https://www.linkedin.com/pulse/critical-role-artificial-intelligence-machine-big-data-rodriguez/
Bain, W. L. (2022, January 13). Subscribe to TDWIBecome a TDWI MemberBecome a part of the TDWI Research PanelSpeak at TDWI EventsBecome a TDWI Research FellowShowcase your Data & AI SolutionsMachine Learning to power the future of streaming Analytics. TDWI. https://tdwi.org/articles/2022/01/13/adv-all-machine-learning-to-power-the-future-of-streaming-analytics.aspx
Comments