How Data Lakes and Data Warehousing are Changing Business Insights
- Minakshi DEBNATH
- May 13
- 4 min read
MINAKSHI DEBNATH | DATE: December 18,2024
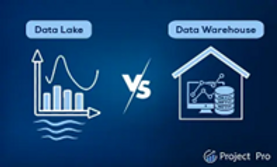
In today's data-driven economy, businesses across all sectors are increasingly relying on data to gain insights, optimize operations, and enhance decision-making. Two critical technologies that enable businesses to extract value from their vast amounts of data are data lakes and data warehouses. While these terms are often used interchangeably, they serve distinct purposes and together contribute to transformative business insights.
The Rise of Data Lakes and Data Warehousing
Organizations today are producing an unprecedented volume of data, with the International Data Corporation (IDC) predicting that the global data sphere will reach 175 zettabytes by 2025 (Reinsel et al., 2018). As businesses strive to harness this data, technologies like data lakes and data warehouses have become indispensable.
A data warehouse is a centralized repository optimized for analyzing structured data from transactional systems and operational databases. On the other hand, a data lake is a scalable storage solution that can handle vast quantities of structured, semi-structured, and unstructured data from diverse sources, typically in raw format.
Key Differences Between Data Lakes and Data Warehouses
Data Structure:
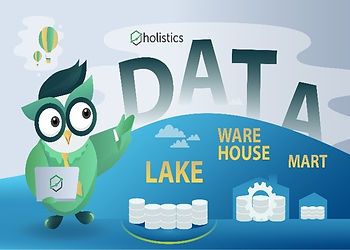
Data Warehouses: Primarily store structured data, often in pre-processed and organized formats that facilitatequick querying and analysis.
Data Lakes: Store all types of data, including raw and unstructured data such as images, videos, social media content, and sensor data.
Processing:
Data Warehouses: Use schema-on-write; data must be cleaned and transformed before ingestion, which ensures high performance for analytics.
Data Lakes: Employ schema-on-read, allowing raw data to be ingested immediately and analyzed later, offering greater flexibility.
Cost: Data lakes, often built on affordable cloud storage solutions (e.g., Amazon S3, Azure Data Lake), are typically more cost-effective than traditional data warehouses, which require higher upfront investments for structured data processing.
Use Cases:
Data Warehouses: Best suited for business intelligence (BI), reporting, and operational analytics where structured data is critical.
Data Lakes: Ideal for big data analytics, machine learning (ML), and exploratory research, where the flexibility to process diverse data formats is essential.
Below are some key areas where this transformation is taking place:
Scalable and Comprehensive Data Management

Traditional data warehousing solutions often struggled to manage the diversity and sheer scale of modern data. Data lakes provide businesses with the ability to collect multi-source data at scale without worrying about schema definitions upfront. This allows organizations to: Aggregate data from IoT devices, social media feeds, transactional systems, and customer interactions. Use big data frameworks like Apache Spark and Hadoop for distributed
processing. For example, Netflix uses a combination of data lakes (Amazon S3) and data warehousing (Amazon Redshift) to analyze massive datasets, optimize content recommendations, and improve viewer engagement (AWS Case Studies, 2022).
Enhanced Data-Driven Decision-Making
Businesses are increasingly relying on advanced analytics and AI/ML models to drive decision-making. Data lakes play a crucial role here by providing the raw data required to train machine learning models, while data warehouses help businesses monitor KPIs and generate actionable insights through dashboards. For instance, healthcare organizations are leveraging data lakes to analyze unstructured patient data (e.g., medical records and images) for disease detection, while data warehouses are used to track hospital performance metrics like readmission rates and patient satisfaction.
Real-Time Insights and Business Agility
The ability to combine real-time data ingestion with fast querying has led to more responsive business operations. While traditional data warehouses focus on historical analysis, modern businesses are adopting streaming analytics capabilities within data lakes. For example, a retailer can use a data lake to monitor real-time transactions and customer behavior across physical and digital channels, enabling quick inventory adjustments, personalized marketing, and improved supply chain efficiency.
Improving Customer Experience
Organizations can now derive deeper insights into customer preferences by analyzing both structured (e.g., purchase history) and unstructured data (e.g., customer feedback, social media comments). A data lake provides the flexibility to store raw customer data, which can be further refined in a data warehouse for targeted analysis. For instance, Starbucks uses data lakes and warehouses to analyze purchase trends, customer loyalty data, and location-specific preferences to deliver personalized offers and enhance customer engagement (Forbes Insights, 2021).
Modern Architecture: Combining Data Lakes and Warehouses. The boundaries between data lakes and data warehouses are increasingly blurring, leading to modern hybrid architectures that combine the strengths of both. Technologies like Snowflake, Google BigQuery, and Azure Synapse Analytics now allow businesses to query data lakes directly using SQL-like languages while maintaining the performance benefits of data warehouses.
A typical modern architecture includes:
Data Ingestion: Collect data from multiple sources into a data lake (e.g., Amazon S3).
Data Transformation: Use tools like Apache Spark, Talend, or AWS Glue to clean and prepare the data.
Data Warehousing: Move processed data to a warehouse (e.g., Redshift, BigQuery) for BI and reporting.
Analytics and AI: Run machine learning or advanced analytics on raw data within the lake.
Conclusion
The evolution of data lakes and data warehousing is revolutionizing how businesses manage, analyze, and derive insights from their data. While data warehouses remain essential for structured, performance-critical analytics, data lakes offer unparalleled flexibility for big data processing and machine learning. Together, they enable organizations to adopt a holistic approach to data-driven decision-making, leading to improved business agility, operational efficiency, and customer satisfaction. By investing in scalable, integrated data solutions, businesses can gain a competitive edge in the digital economy, turning raw data into valuable insights that drive innovation and growth.
Citation/References:
AWS Case Studies. (2022). Netflix: Transforming Entertainment with Data-Driven Insights. Retrieved from
Forbes Insights. (2021). How Starbucks Leverages Big Data Analytics to Enhance Customer Experience. Retrieved from
https://www.forbes.com/insights/starbucks-big-data-case-study/
Snowflake Inc. (2022). Combining Data Lakes and Warehouses: Modern Data Architectures. Retrieved from
https://www.snowflake.com/blog/data-lakes-and-data-warehouses/
Image Citations
Data Lake vs Data Warehouse - Working Together in the Cloud
https://www.projectpro.io/article/data-lake-vs-data-warehouse/463
Data Lake vs Data Warehouse vs Data Mart
https://www.holistics.io/blog/data-lake-vs-data-warehouse-vs-data-mart/
Data Lake vs. Data Warehouse: What’s the Difference
https://blog.nashtechglobal.com/data-lake-vs-data-warehouse-whats-the-difference/
Comentarios