The Future of Edge AI: Combining Intelligence with Low Latency
- Arpita (BISWAS) MAJUMDAR
- 7 days ago
- 4 min read
ARPITA (BISWAS) MAJUMDER | DATE: DECEMBER 26, 2024
In an era where immediacy and intelligence are paramount, Edge Artificial Intelligence (AI) stands at the forefront of technological innovation. By bringing computational power closer to data sources, Edge AI combines advanced intelligence with low latency, enabling real-time decision-making across various industries.
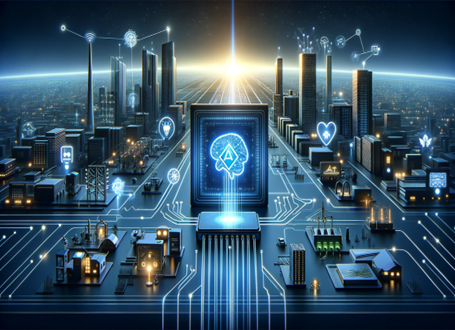
Understanding Edge AI
Edge AI involves deploying AI algorithms directly on devices at the network's edge—such as smartphones, IoT devices, and autonomous vehicles—rather than relying solely on centralized cloud servers. This approach allows data to be processed locally, reducing the time it takes to analyse information and respond accordingly.
Advantages of Edge AI
Reduced Latency: Processing data locally eliminates the delays associated with transmitting data to and from distant servers, enabling instantaneous responses crucial for applications like autonomous driving and industrial automation.
Enhanced Privacy and Security: Local data processing minimizes the need to send sensitive information over networks, reducing exposure to potential breaches and enhancing user privacy.
Bandwidth Optimization: By handling data at the edge, the amount of information transmitted over networks is significantly reduced, conserving bandwidth and lowering costs.
Reliability: Edge AI systems can operate independently of internet connectivity, ensuring consistent performance even in areas with limited or intermittent access.
Applications of Edge AI

Autonomous Vehicles: Self-driving cars require real-time data analysis from sensors and cameras to navigate safely. Edge AI facilitates immediate processing of this data, enabling quick decision-making without relying on cloud connectivity.
Healthcare: Wearable devices equipped with Edge AI can monitor vital signs in real-time, detect anomalies, and alert users or healthcare providers promptly, enhancing patient care and response times.
Industrial Automation: In smart factories, Edge AI analyses data from machinery to predict maintenance needs, optimize operations, and prevent downtime, leading to increased productivity and cost savings.
Smart Cities: Edge AI enables real-time monitoring and management of urban infrastructure, including traffic control, energy distribution, and public safety systems, contributing to more efficient and liveable urban environments.
Challenges in Implementing Edge AI
Although Edge AI offers numerous advantages, it also presents several challenges that need to be tackled effectively:
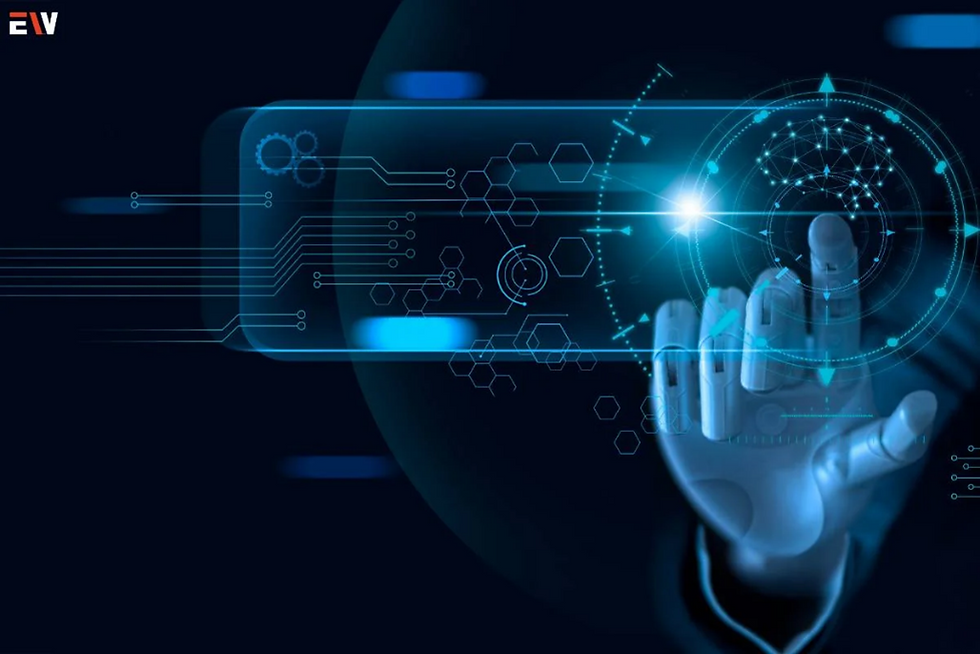
Hardware Constraints: Edge devices often have limited processing power, memory, and energy resources, making it challenging to run complex AI models. Developers must optimize algorithms to balance performance and resource consumption, ensuring efficient execution on edge devices.
Scalability: Deploying and managing AI models across numerous devices can be complex, requiring robust frameworks for updates and maintenance. The lack of hardware standards further complicates scalability, as integrating diverse devices into a cohesive system poses significant challenges.
Interoperability: Ensuring seamless communication and integration between diverse devices and systems is essential for the cohesive functioning of Edge AI applications. The absence of standardized protocols can hinder interoperability, necessitating the development of universal standards to facilitate seamless integration.
Security Concerns: While local processing enhances privacy, edge devices can still be vulnerable to physical tampering and cyber-attacks. Implementing robust security measures is crucial to protect data integrity and prevent unauthorized access.
The Future of Edge AI
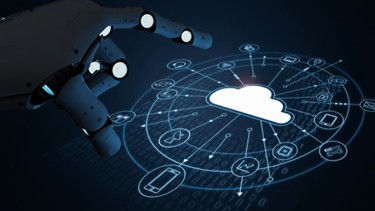
The convergence of AI with edge computing is set to revolutionize industries by delivering intelligent solutions with unprecedented speed and efficiency. Advancements in hardware, such as specialized AI chips for edge devices, and the development of more efficient algorithms are propelling this evolution. The integration of 5G technology further enhances Edge AI capabilities by providing high-speed, low-latency connectivity, enabling more sophisticated applications, particularly in IoT and smart city scenarios.
As these technologies mature, we can anticipate a proliferation of applications that leverage Edge AI to offer smarter, faster, and more responsive experiences in our daily lives. The ongoing research and development in this field aim to address current challenges, paving the way for more robust and scalable Edge AI solutions.
Conclusion
Edge AI represents a significant leap forward in the quest for real-time, intelligent data processing. By combining advanced AI capabilities with low-latency processing at the network's edge, it opens up new possibilities across various sectors, from autonomous vehicles to smart cities. While challenges remain, the continuous advancements in technology and infrastructure promise a future where Edge AI plays a pivotal role in shaping the intelligent systems of tomorrow.
Citations/References
Up, R. (2024, November 14). Edge Computing — reducing latency in future AI systems. Medium. https://rocketmeup.medium.com/edge-computing-reducing-latency-in-future-ai-systems-679ed3b8910a
Revisiting Edge AI: Opportunities and challenges. (n.d.). IEEE Journals & Magazine | IEEE Xplore. https://ieeexplore.ieee.org/abstract/document/10621659
Staff, W. (2024, April 8). Chapter 11: The Future of Edge AI | 2024 State of Edge AI Report. Wevolver. https://www.wevolver.com/article/2024-state-of-edge-ai-report/the-future-of-edge-ai
Pandit, B. (2024, August 6). What is Edge AI? How it works, applications, challenges. https://www.datacamp.com/blog/edge-ai
Team, E. I. (2024, July 19). The convergence of edge AI and cloud: Making the right choice for your AI strategy. Edge Impulse. https://www.edgeimpulse.com/blog/edge-ai-vs-cloud-computing-making-the-right-choice-for-your-ai-strategy/
Boesch, G. (2024, December 6). Edge AI – Driving Next-Gen AI applications in 2024. viso.ai. https://viso.ai/edge-ai/edge-ai-applications-and-trends/
Evanczuk, S. (2023, September 12). Tackling edge AI challenges. Embedded. https://www.embedded.com/tackling-edge-ai-challenges/
Sinha, S. (2024, September 26). The top 6 edge AI trends—as showcased at Embedded World 2024. IoT Analytics. https://iot-analytics.com/top-6-edge-ai-trends-as-showcased-at-embedded-world-2024/
Medici, G. (2024, July 15). Syntiant: Overcoming the challenges of Edge AI — Syntiant. Syntiant. https://www.syntiant.com/news/syntiant-overcoming-the-challenges-of-edge-ai
Zharovskikh, A. (2024, December 1). Cutting edge AI. InData Labs. https://indatalabs.com/blog/cutting-edge-ai
Image Citations
(27) Embracing the future of Edge AI: Opportunities, Challenges, and Strategies | LinkedIn. (2024, March 26). https://www.linkedin.com/pulse/embracing-future-edge-ai-opportunities-challenges-eugene-terente-xutyf/
Earney, S. (n.d.). A comprehensive guide to edge AI. https://xailient.com/blog/a-comprehensive-guide-to-edge-ai/
Wired, E. (2024, January 25). Power of Edge AI: Bridging the gap between data and real-time processing | Enterprise Wired. Enterprise Wired. https://enterprisewired.com/power-of-edge-ai-bridging-the-gap-between-data/
Preetipadma, & Preetipadma. (2020, June 29). How Edge AI is a Roadmap to Future AI and IoT Trends? Analytics Insight. https://www.analyticsinsight.net/artificial-intelligence/edge-ai-roadmap-future-ai-iot-trends
About the Author
Arpita (Biswas) Majumder is a key member of the CEO's Office at QBA USA, the parent company of AmeriSOURCE, where she also contributes to the digital marketing team. With a master’s degree in environmental science, she brings valuable insights into a wide range of cutting-edge technological areas and enjoys writing blog posts and whitepapers. Recognized for her tireless commitment, Arpita consistently delivers exceptional support to the CEO and to team members.
Comments